Better photonics with machine learning
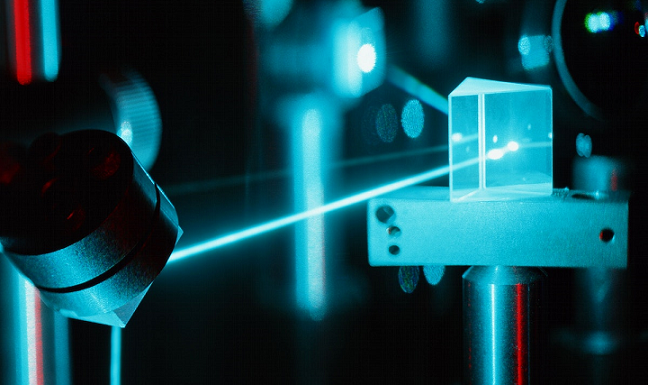
Published on 24/01/2023
From optics to photonics
Optics is the field of physics studying the behaviour of light and its interaction with matter. In modern theories of physics, light is considered either as a wave or as quantum grains called photons. These photons give their name to a new field, photonics, which study light-matter interactions and seeks to tailored light at the nanoscale.
Michaël Lobet is a “photonician”, like he calls himself, in the laboratory of solid-state physics (LPS) at the University of Namur.
Michaël Lobet (UNamur): “We study the propagation, emission, absorption or reflection of light. In the past, these phenomena were related to the fields of optics, now we rather have a quantum vision of the light.”
Applications of photonics are numerous: from telecommunications using optical fibre, which allows the transmission of information close to the speed of light, to the production of photovoltaic energy or also photocatalysis (the activation of chemical reaction from light) for the treatment of air pollutants.
Numerical simulations, at the junction between theory and experiments
For Michaël Lobet, the research work is divided into two theoretical parts (analytical study and numerical simulations) and the experimental part. Michaëls work is mainly theoretical:
Michaël Lobet: “In photonics, there is a whole set of concepts that can be studied with paper and pencil. But real systems are usually much more complex, and it's necessary to use numerical methods. We solve the equations of electromagnetism numerically to study structured materials in one, two or three dimensions. We have many numerical tools at our disposal in our toolbox of numerical photonician and we have long expertise with these tools in the solid-state physics laboratory.”
But for Michaël Lobet, it is important to stay in touch with the experimental side of the research. He is in constant interaction with experimentalists, either to propose new experiments, explore a new concept or conversely, propose a model to explain an experimental reality.
Machine learning to help photonics research
A realistic physical system is very complex and depends on many parameters. The physical properties of this system will depend on all these parameters, and optimizing it to obtain the desired properties is not an easy task.
Michaël Lobet: “Let's take the example of a photovoltaic cell, there are electrodes, then an absorbing layer which can be structured, for example with small beads. These beads can have different radii, the number of layers of beads can change, the total thickness varies etc. This gives an extremely large number of possible configurations.”
The advantage of numerical simulations is obvious here since it would be simply impossible to realize experimentally so many different systems to check which configuration provides the best yield. However, even numerically, some problems remain very complex to tackle, and it is necessary to find strategies to solve them efficiently. This is where machine learning and supercomputers come into play.
Michaël Lobet: “The work can be subcontracted by supercomputers that will split the problem to produce an interesting solution. The contributions of machine learning in photonics are quite important. Even if, eventually, it is still the physicist’s job to understand why this solution is optimal.”
From photovoltaics optimization…
To solve these numerical problems, Michaël surrounds himself with other researchers, more specialized in machine learning. For example, Alexandre Mayer has developed a genetic algorithm to optimize physical systems according to the desired properties.
Alexandre Mayer: “We test fifty different configurations, we then select the best configurations, we cross their characteristics, we introduce some mutations, and we start again until we obtain the best solution for the considered problem.”
This requires working on supercomputers.
Alexandre Mayer: “I work on Hercules (the supercomputer of UNamur), Dragon (UMons) and sometimes Tier-1 (Cenaero) to run these calculations in parallel. A simulation can last from a few hours to one or two days depending on the application.”
Using this genetic algorithm, Michaël Lobet and Alexandre Mayer have studied perfect absorbers, artificial materials that absorb nearly 100% of the light on a large part of the light spectrum [1].
Michaël Lobet: “I already worked on perfect absorbers during my thesis. It was a nanometric pyramidal structure composed of 20 layers of gold. The optimizations "by hand" gave an absorption of 98% between 200 nm and 5.6 µm. But the structure was impossible to realize experimentally because of the large number of layers. So, we repeated the simulation now using the genetic algorithm of Alexandre Mayer. This allowed us to reduce the number of layers of the pyramids down to 3 while keeping a perfect absorption (99,8%) over a broad range.”
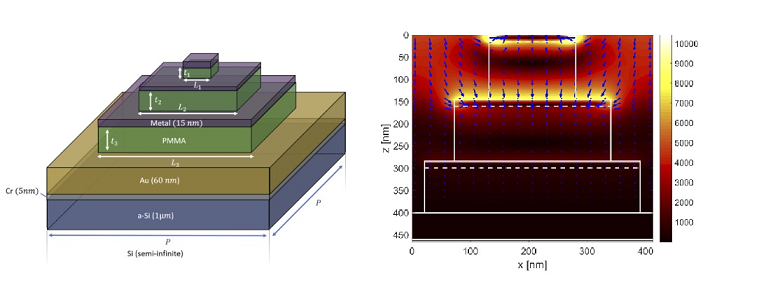
This algorithm also helped to find a better design for photovoltaic cells with perovskite, a material that is intended to replace or complement silicon in solar cells. This work was done in collaboration with the CESAM-GREENMAT laboratory of the University of Liège (ULg), which produces solar cells. They understood that they had to structure the material to obtain better light trapping. With the optimization of this structure, they showed how to improve the yield of the cells [2].
… to exoplanet observation
On another side, still related to photonics and machine learning, Ph.D. student Nicolas Roy uses state-of-the-art neural networks such as the U-Net architecture, which permits to generate an image from another image. He uses this architecture for physical system optimization problems with the help of a genetic algorithm:
Nicolas Roy: “For example, a numerical simulation code for photonics will take us from a permittivity map (a known property of the system’s components) to the leakage map (the property of the system we want to know). These simulations, which can take up to a day of computation for high-fidelity, are not practical for optimization with the genetic algorithm. It is therefore replaced by U-Net, which, once trained, will be much faster to obtain accurate predictions”.
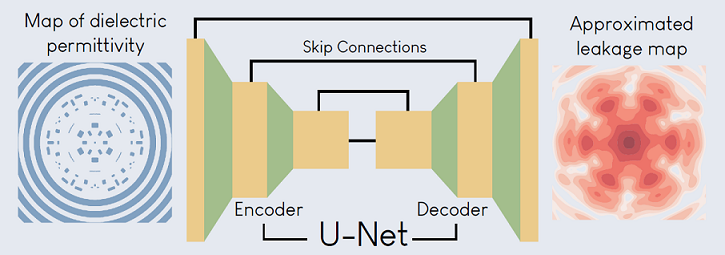
These calculations are done on CECI’s supercomputers:
Nicolas Roy: “The general program runs on Nic5 (ULg). Only the neural network training is done on Hercules, on which we can use GPUs.”
Nicolas Roy and Michaël Lobet's most recent work is on coronography in collaboration with the team of Olivier Absil of the STAR Institute of the ULg. The basic idea of coronography is to observe what happens in the periphery of distant stars by attenuating the star's light.
Michaël Lobet: “The problem is that the luminosity of the star is so great that we can’t spot its companions. An analogy is to try to spot the light emitted by a firefly that turns around a lighthouse. It is thus necessary to use optical techniques to hide the light of the headlight, this is the role of the coronograph.”
The team of Olivier Absil has been developing diffraction gratings for more than 20 years. These gratings enable to divert the light of the star towards the outside and concentrate the light of the exo-planet at the focal point of the telescope. These diffraction gratings depend on many parameters that can be optimized.
Michaël Lobet: “This is where we used the machine learning techniques developed by Nicolas to go faster in the optimizations. We found optimums for these structures, which will eventually be manufactured in collaboration with the University of Uppsala in Sweden. These coronographs will then in the future be embarked on board of satellites to make imaging of exoplanets.”
With the rapid growth of machine learning and AI, no doubt that such use of HPC will be of great interest for future research not only in photonics but in all research domains.
[1] A. Mayer et al., Opt. Express 2022, 30, 1167– 1181
[2] Nathan Daem et al., ACS Appl. Nano Mater. 2022, 5, 9, 13583–13593
Dr. Michaël Lobet
Michaël Lobet obtained his PhD in 2016 in the Laboratory of Solid-State Physics (LPS) at the University of Namur, with Pr. L. Henrard and Pr. O. Deparis. He then pursued post-doctoral research in the laboratory of Pr. E. Mazur in Harvard before joining Pr J. Loicq at the “Centre spatial de Liège” (ULg). Since October 2022, he is a Research fellow of the FNRS (Fonds National de la Recherche Scientifique) in the LPS. His research focuses on theoretical optics and photonics of photonic crystals and 2D materials.
Dr. Alexandre Mayer
Dr. Alexandre Mayer is a Research Fellow of the FNRS (Fonds National de la Recherche Scientifique) since 2002. He obtained a PhD in physics in 1998 at the University of Namur, under the supervision of Jean-Pol Vigneron. He was postdoc at the Pennsylvania State University in 2001, where he worked on field electron emission with Paul Cutler & Nick Miskovsky. He is an expert in high-performance computing and genetic algorithms. His current interests include machine learning & inverse design techniques in optics.
Nicolas Roy
Nicolas Roy obtained his master’s thesis in 2018 on numerical methods for photonics under the supervision of Pr. O. Deparis and a Master's degree in Mathematics the following year with Pr. T. Carletti, working on metaheuristics. He is currently working on his PhD, delving into the intersection of machine learning and photonics under the guidance of his supervisor, Alexandre Mayer, and Charlotte Beauthier from Cenaero .